Generative AI, A New Paradigm in Artificial Intelligence
- Summer Atlantic
- May 13, 2024
- 7 min read
Updated: May 14, 2024
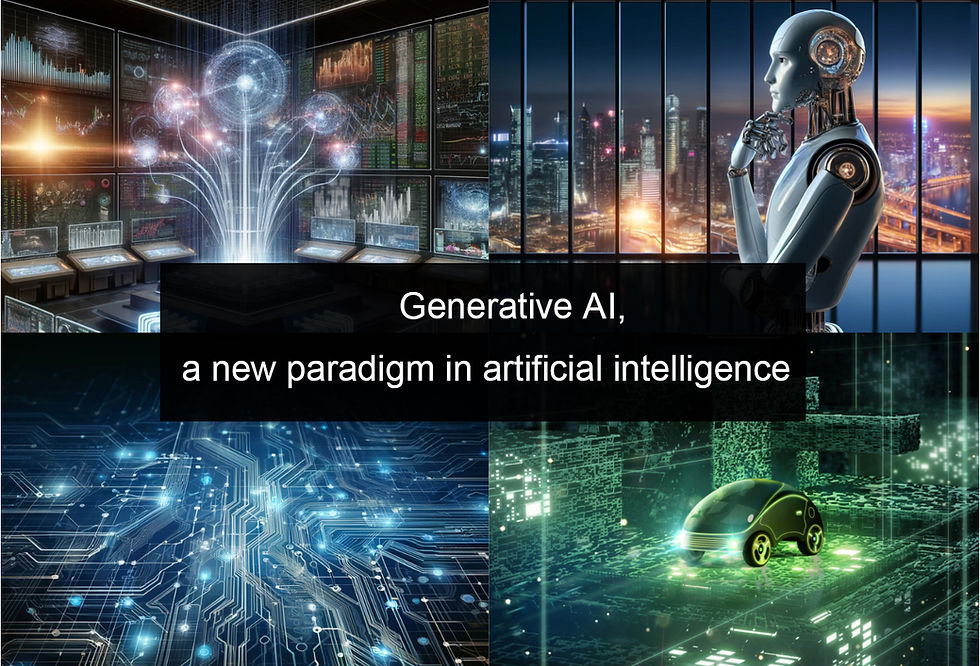
Generative AI, with its remarkable human-like creativity, stands at the forefront of AI technology. It's revolutionizing fields such as content generation, textual analysis, customer service, and coding. The productivity level is more than 4.4 times of human labor.
Generative AI's rapid development and integration across various sectors can be attributed to significant advancements in models, increased computing power, and the growth of supportive ecosystem.
1. Model. The release of the Transformer model in 2017 and ChatGPT in 2020, along with continuous breakthroughs in multimodal ecosystems and large-scale visual models, signify a major leap in generative AI in the text domain.
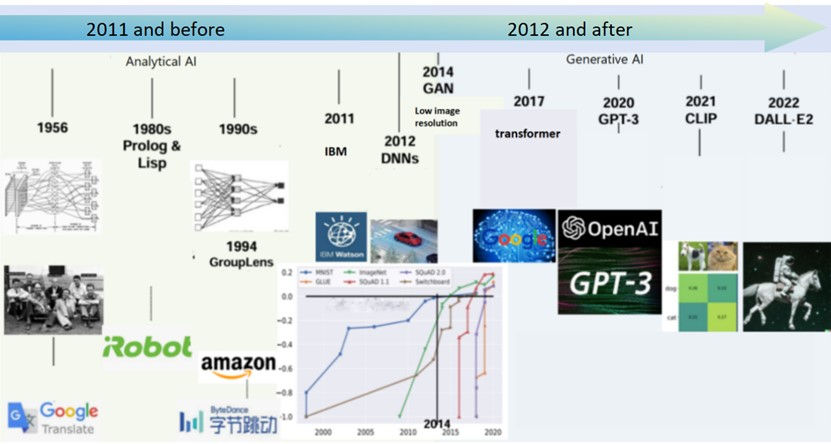
2. Computing Power. Computing infrastructure becomes faster and cheaper. NVIDIA is enhancing its GPU performance, while leading tech companies such as Microsoft, Amazon, Google, and Facebook are boosting their investments in AI computing cloud services and the development of their own AI chips.
3. Ecosystem. The refinement of the AI component layer (AI Stack) and the evolution of industrial division of labor provide full lifecycle support for AI applications in model training, data integration, application development, and deployment.
According what Gartner said, from replacing repetitive tasks to generating innovative content, AI's capabilities span a wide range. It also predicts that, by 2023, 20% of organizations will be using AI services; and by 2025, AI's contribution to enterprise revenue growth will reach 10%. However, due to various reasons such as a lack of awareness in identifying business opportunities, organizations may lose out on AI-driven growth opportunities.
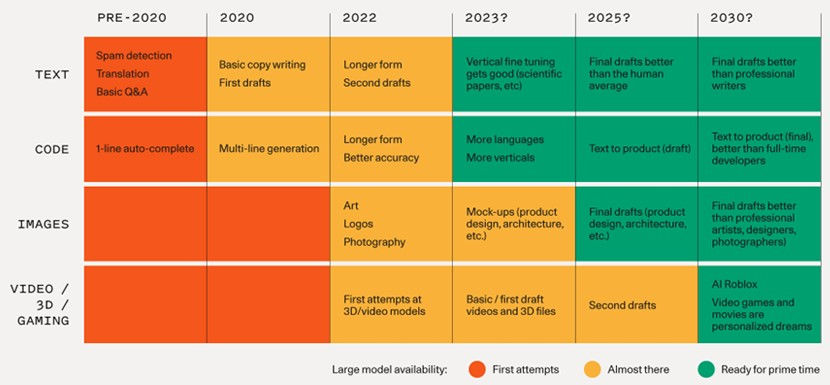
Application Scenarios
Finance
Over the past few decades, the financial industry has undergone a thorough transformation with the support of technology. For instance, shopping no longer requires cash or card swiping, and the more transactions there are, yet the less queuing there is inside banks.
In practical applications, large model providers with specialized risk prevention and control technologies have indeed become the primary partners of financial institutions both domestically and internationally. For example, many financial companies around the world have chosen OpenAI on Microsoft's Azure as their partner. Its AI solutions are capable of detecting synthetic voices, helping HSBC save over $400 million in costs related to identity theft and fraud prevention. Also, Swift collaborates with Microsoft in anti-money laundering, using Azure confidential computing and large AI models to detect fraudulent patterns in interbank fund flows, without needing to access the data of bank customers.
Healthcare
Generative AI supports Interactive Voice Response (IVR) processes by cross-referencing patients' medical and claims records, thereby creating a more personalized user experience while also reducing the volume of calls that need to be handled by staff. It can also summarize patients' past treatments to create future management plans for subsequent follow-up. Furthermore, by analyzing customer texts and trends, such as the most common claims and types of medical visits, moments of greatest customer dissatisfaction, and questions that confuse customers the most, it helps healthcare providers to identify the “shortest stave”.
Manufacturing
In mining, it is necessary to prevent premature equipment failure, avoid costly repairs and replacements, and extend the lifespan of assets. Generative AI, by considering operational factors such as equipment usage, production requirements, and maintenance costs, can recommend the most cost-effective maintenance plans for enterprises. It can also analyze equipment usage and performance data to minimize downtime, thereby helping to optimize maintenance schedules.
Culture and entertainment
In content creation, generative AI is used for scriptwriting, original dialogue design, and character generation. In media, it encompasses content tag extraction and inter-frame restoration. In the field of communications, it involves news production and generating video materials. Here is an application example.
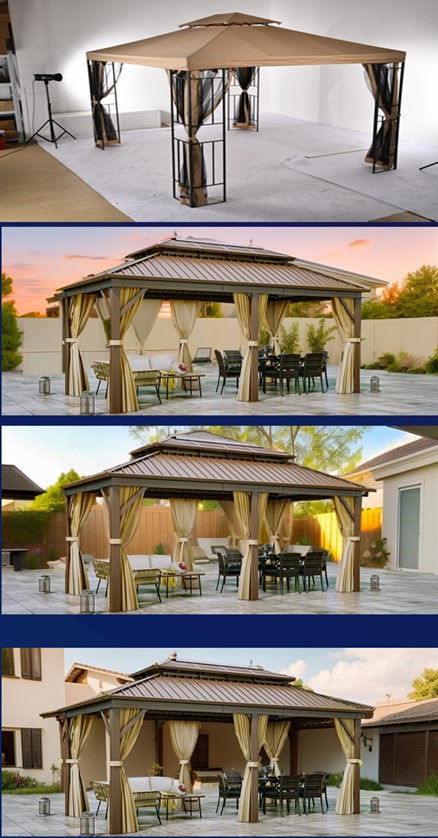
Service Provider
Amazon
Amazon Titan: Used for text summarization, text generation, classification, open-ended question answering, information extraction, embedding, and search.
AI21 Labs Jurassic-2 Multilingual LLM: Used for generating text in various languages.
Anthropic Claude 2: Based on Constitutional AI and harmlessness training, used for insightful conversation, content creation, complex reasoning, creativity, and code writing LLM.
Stability AI Stable Diffusion: Generates unique, realistic, high-quality images, artwork, logos, and designs.
Cohere Command + Embed: Text generation model for business applications and embedding model for search, clustering, or classification in over 100 languages.
Here is an example of an AWS architecture.
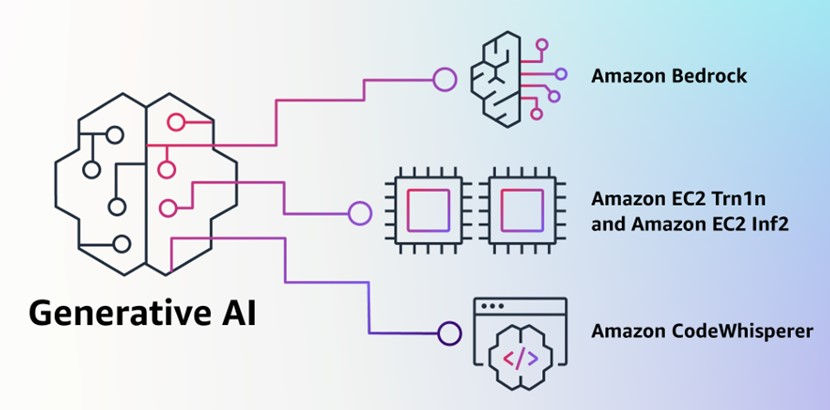
Azure
Azure integrates OpenAI models into the cloud services domain. This allows more businesses to deploy using advanced AI models, including GPT-3.5, Codex, and DALL·E 2.
Since 2000, Microsoft's development has roughly gone through three phases: 1) 2000s: In 2000-2001, Microsoft launched Windows 2000/XP but missed the development opportunity of the mobile internet, resulting in a significant drop in stock price; 2) 2010s: In 2008, Microsoft launched Azure, laying out its cloud computing strategy, and in 2015, it open-sourced and adapted to Linux, which rapidly boosted its performance; 3) Since 2019: Microsoft has been focusing on the field of artificial intelligence, including investing in AI companies such as OpenAI and Nuance. In 2023, Microsoft announced an additional investment in OpenAI, and their partnership entered its third phase.
Existing challenges
Model providers face a series of key challenges in the model training phase.
1. Big Data: The data used for training large models requires a greater volume, higher quality, and stronger security. Tasks such as ensuring privacy is effectively protected without compromising the data's utility present significant challenges.
2. Large Parameters: Large models often have parameters in the trillions. During training, file storage, reading, and writing, as well as cluster stability, demand high architectural requirements. 3. High Computing Power: It is necessary to ensure computational performance and stability while reducing overall costs.
Developers are responsible for implementing technology at scale, facing the following challenges:
1. Model Selection and Evaluation. The application scenarios in actual businesses are complex and diverse, necessitating consideration of compatibility issues for different vendor models and deployment environments.
2. Solving Data Silos. Large models make the integrated use of multimodal data possible. This requires developers to deploy proper APIs, data warehouses and pipelines to allow for the free flow of data across different departments or systems within an organization, leveraging the full potential of the whole data assets.
Users face the following challenges:
1. Privacy Concerns. Users worry that using third-party models and the cloud may lead to the leakage of core data.
2. Cost Control. There is a desire to reduce the costs of infrastructure and paid services while ensuring high concurrency and low latency.
3. Personnel Training. There is a shortage of enterprise-related skilled personnel for new tools.
Policies
The U.S.
In June 2021, the U.S. Government Accountability Office defined accountability practices around principles such as governance, data, performance, and monitoring in "Artificial Intelligence: Accountability Framework for Federal Agencies and Other Entities."
In October 2022, the White House Office of Science and Technology Policy published a white paper titled "Blueprint for an AI Bill of Rights-Making Automated Systems Work for the American People," which outlined five fundamental principles of artificial intelligence.
In January 2023, the National Institute of Standards and Technology, a subsidiary of the U.S. Department of Commerce, released the "Artificial Intelligence Risk Management Framework" and its accompanying user guide, which defined the risks associated with artificial intelligence.
China
Generative artificial intelligence services targeting mainland China must simultaneously meet the requirements of the "Algorithm Recommendation Regulations," the "Deep Synthesis Regulations," and the "Generative Methods Regulations."

APAC Potential
According to survey from Infosys, APAC companies have collectively spent $1.4 billion more than Europe on generative AI in the past year but still lag behind North American firms. In the future, APAC companies plan to increase their spending on generative AI by over 140% to reach $3.4 billion by 2024. This anticipated growth rate outpaces Europe's forecasted rate of 115% and are expected to further close the spending gap with North America, potentially doubling the expected growth rate and extending their lead in the region.

China, with its highly developed technology sector, is expected to lead the APAC region.

A study encompassing 12 sectors spanning Australia, China, India, Japan, New Zealand, and Singapore shows that financial services, high tech, insurance, and manufacturing sectors report a substantially greater number of initiatives generating value compared to almost all other industries surveyed. Healthcare, however, emerges as the sole industry significantly trailing behind others in both implementation efforts and the creation of business value.

According to a report by Salesforce, which collected insights from over 4,000 IT leaders across 28 countries, including 1,400 from APAC, 88% highlights the growing importance of generative AI in the region's technological landscape.
Let’s take a deeper dive into it. The latest Asia Pacific Readiness Index by Salesforce evaluates the AI readiness of 12 countries in the region.
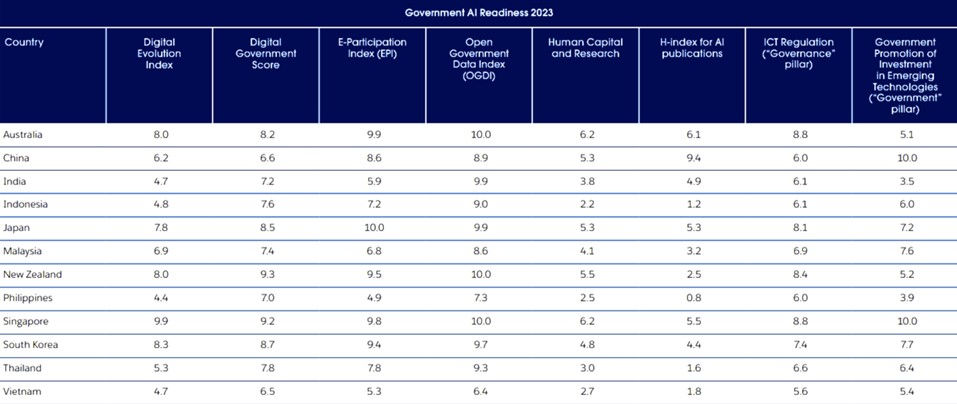
In the Asia-Pacific region, nations like Singapore, China, Japan, South Korea, and Australia have implemented policies aimed at leveraging generative AI opportunities. However, Vietnam and the Philippines, which rank lower. Despite increased government readiness overall, differences between high and low scorers remain consistent. Vietnam experienced the smallest increase. Actually Vietnam invests heavily in AI, but progress is hindered by a lack of AI talent and expertise.
To conclude, the success of countries in harnessing the potential of generative AI relies on robust institutional, infrastructural, organizational, and ethical frameworks.
In Australia, it is projected that generative AI could potentially add approximately AUD 115 billion (equivalent to around $76 billion) annually to the economy by 2030, contingent upon its adoption rate and how smoothly workers transition to alternative roles. Similarly, in Japan, generative AI is forecasted to release productive capacity equivalent to approximately JPY 148.7 trillion (about $1.1 trillion).
"In accordance with the survey results, it's widely agreed that generative AI technology is poised to significantly enhance both employee productivity and customer satisfaction," remarked Tony Ng, Managing Director of Greater China at Adobe.
The potential of GenAI can be shown with a specific application example. Organizations like Singapore’s National University Health System (NUHS) are forging partnerships with technology giants like Amazon Web Services (AWS) to pilot GenAI solutions in healthcare. Their focus lies on automating tasks such as creating patient discharge summaries, with an emphasis on precision, security, and thorough testing of GenAI applications.
While some APAC organizations are willing to pay a premium for products or services integrated with GenAI, a considerable percentage remains uncertain, underlining the necessity for robust financial operations (FinOps) to efficiently manage GenAI workload expenses. Successfully navigating the complexities of GenAI adoption requires a strategic approach. Bhargs Srivathsan of McKinsey emphasizes the importance of selecting a suitable model tailored to organizational needs, cautioning against overspending on unnecessarily complex models. The judicious management of GenAI usage can significantly influence the feasibility and success of its integration into business frameworks.
In conclusion, the potential of Generative AI (GenAI) is significant and multifaceted. It can revolutionize business operations and pave the way for a more efficient and dynamic future.
Comments